The world of genomics is changing fast, and artificial intelligence (AI) is leading the charge. With its ability to process vast amounts of complex genetic data, AI is helping researchers uncover patterns and make predictions that were once impossible. As a result, we are seeing breakthroughs in precision medicine, where treatments are tailored to individual patients, and discoveries in genetic diseases that could transform healthcare.
Moreover, deep learning, a powerful type of AI, allows scientists to analyze DNA sequences and gene functions with incredible accuracy. This technology is not just advancing research—it’s saving lives. So, let’s explore how AI is revolutionizing genomics and opening doors to a healthier, more personalized future.
Understanding the Basics of AI in Genomics
Artificial intelligence (AI) is transforming genomics, but it’s important to understand that AI is not a magic wand. It doesn’t instantly solve problems or make discoveries without effort. Instead, AI relies on complex statistical and mathematical algorithms that analyze data, identify patterns, and generate insights. While it is powerful, it requires high-quality data and careful implementation to work effectively.
When you hear about AI in genomics, think of it as a tool—a highly advanced one—that helps researchers process and understand massive amounts of genetic information. Together, we can use AI to uncover insights that were previously impossible to find, but this requires a clear understanding of how it works and what it can do.
What Is AI in Genomics?
AI refers to systems that learn from data and use that knowledge to make decisions or predictions. In genomics, this means analyzing DNA, RNA, and other biological data to find genes, predict diseases, and study genetic variations. However, AI is not a replacement for human expertise. It enhances our ability to interpret data by doing what humans cannot: processing billions of data points quickly and uncovering patterns that would take us years to identify.
Machine Learning vs. Deep Learning
AI in genomics often uses two main approaches: machine learning (ML) and deep learning (DL).
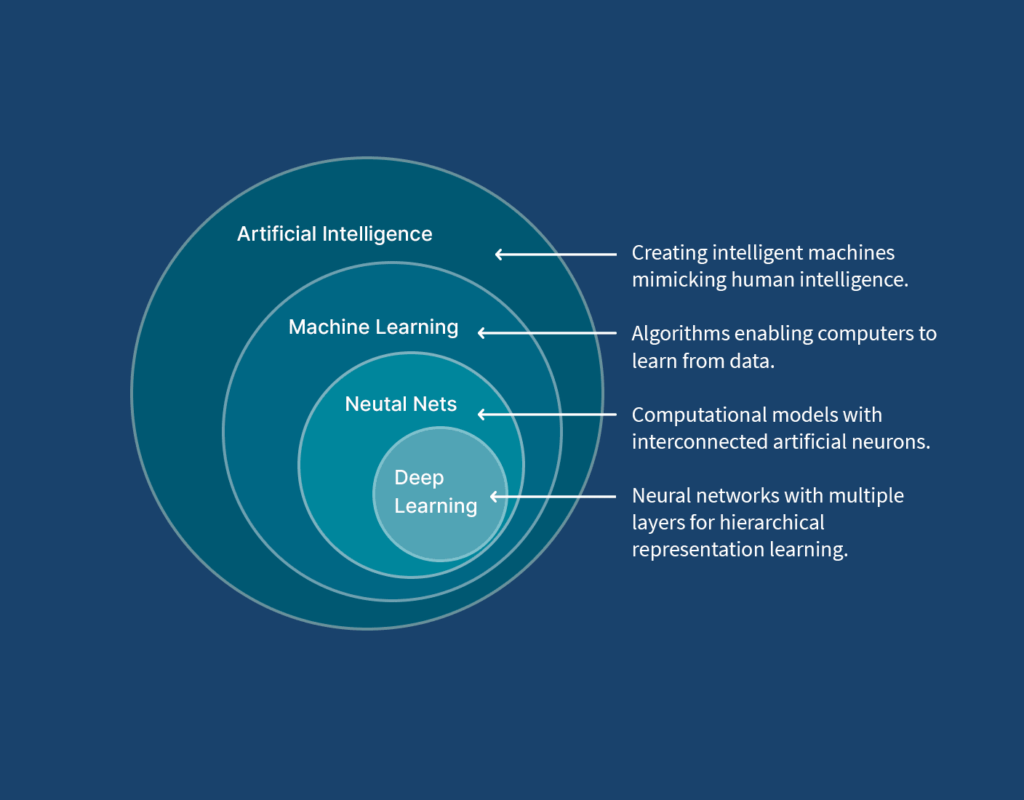
- Machine Learning: This approach involves algorithms that learn from data and apply those lessons to tasks. For example, ML can classify genetic mutations as harmful or benign by learning from labeled datasets.
- Deep Learning: This is a more advanced form of ML that uses neural networks with multiple layers. It excels at processing complex and unstructured data, such as DNA sequences. Unlike simpler methods, DL models can find deeper patterns, such as how a specific mutation influences disease risk.
Why AI Is Needed in Genomics
Genomics generates enormous amounts of data, which can be overwhelming to analyze manually. Modern sequencing technologies produce terabytes of information from a single study, making AI essential. With AI, we can:
- Quickly identify disease-causing mutations, speeding up diagnoses.
- Develop personalized treatments for genetic disorders, improving patient outcomes.
- Predict individual responses to medications, enhancing precision medicine.
Without AI, these processes would take years, but AI enables us to analyze data in days or hours.
AI Is Not Perfect
While AI is a powerful tool, it has limitations. It depends on the quality of the data we provide and the way we design the algorithms. If the data is incomplete or biased, the results will reflect those flaws. This is why you should think of AI as a partner—it helps us, but it doesn’t replace careful analysis, critical thinking, or domain expertise.
Current Trends in AI for Genomics
AI continues to evolve, with exciting trends shaping the field. For instance:
- Researchers are using multi-omics approaches, combining genomic, transcriptomic, and proteomic data to gain a holistic view of biological processes.
- Deep learning models are helping predict gene expression under different conditions.
- Cloud-based AI platforms allow collaboration and enable large-scale genomic studies, making research more accessible.
By focusing on the facts and keeping expectations realistic, we can use AI to its fullest potential. It’s not magic, but when applied thoughtfully, it is an incredibly powerful tool that’s already reshaping genomics and healthcare.
Key Applications of AI in Genomics
AI is making significant strides in genomics, opening doors to faster discoveries and more personalized healthcare. Its ability to analyze complex genetic data at unprecedented speed is changing how we approach medicine and research. Let’s explore some of the key applications that are already transforming the field.
Precision Medicine
One of the most exciting uses of AI in genomics is in precision medicine. This approach tailors treatments to an individual’s unique genetic makeup, offering better outcomes and fewer side effects. For example, AI can analyze a patient’s genetic data to predict how they will respond to a specific drug. By doing this, it ensures you receive treatments that are more effective for your body.
Doctors and researchers also use AI to identify genetic markers that indicate a risk for certain diseases. These insights help you take preventative actions, such as lifestyle changes or early medical interventions, to stay healthier for longer.
Predictive Analytics for Genomic Data
AI excels at predictive analytics, using historical and current data to forecast future outcomes. In genomics, this means predicting your risk for diseases based on your genetic profile. For instance, AI can analyze family history and DNA to estimate the likelihood of developing conditions like cancer or diabetes.
These predictions not only help individuals but also assist healthcare providers in creating targeted public health strategies. With AI, we can move from reactive healthcare to a more proactive approach.
Accelerating Gene Sequencing and Analysis
Gene sequencing, which decodes the instructions in your DNA, is a critical part of genomics. However, it’s time-consuming and generates enormous amounts of data. AI accelerates this process by quickly identifying important genetic patterns and anomalies. For example, AI-powered tools can pinpoint mutations linked to rare diseases in a fraction of the time traditional methods require.
By speeding up analysis, AI helps researchers focus on solutions instead of spending months sorting through data.
Early Disease Detection and Risk Assessment
AI’s ability to detect diseases early is a game-changer. For instance, AI can scan genomic data to identify subtle changes that signal the onset of diseases like cancer, even before symptoms appear. This gives you and your healthcare team more time to act and improves your chances of recovery.
Similarly, AI helps assess risks for hereditary diseases. By combining your genetic data with family history and environmental factors, it creates a clearer picture of your overall health risks.
Speeding Up Drug Discovery
Developing new drugs is a lengthy and expensive process, often taking over a decade. AI shortens this timeline by identifying potential drug targets and predicting how new compounds will interact with the human genome.
For example, AI can quickly screen millions of molecules to find those most likely to work against a specific disease. This not only saves time but also reduces costs, making treatments more accessible to those who need them.
These applications of AI in genomics are just the beginning. The next step is understanding the cutting-edge deep learning techniques that drive many of these advancements. In the following section, we’ll dive into how deep learning models like convolutional and recurrent neural networks are reshaping genomic research. Let’s uncover the science behind the magic!
Deep Learning Techniques Transforming Genomic Research
Deep learning is a game-changer in genomic research, powering breakthroughs by analyzing complex data with unmatched precision. These advanced techniques rely on neural networks—mathematical models inspired by the human brain—that extract patterns from data to make predictions and uncover insights. Among the many architectures used in genomics, several stand out for their transformative impact: convolutional neural networks (CNNs), recurrent neural networks (RNNs), graph neural networks (GNNs), and transformers.
You may interested: Deep Learning Architectures for Biologists: A Simple Guide
Convolutional Neural Networks (CNNs)
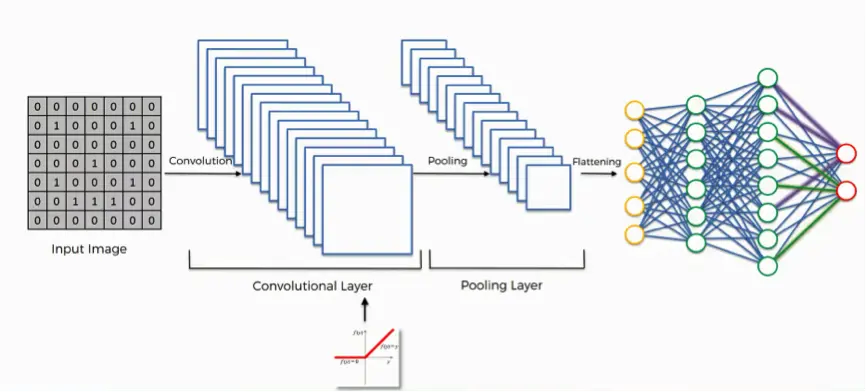
CNNs are experts at recognizing patterns in structured data, making them invaluable for analyzing DNA sequences. They work by scanning genomic data to detect motifs, or recurring patterns, that influence gene regulation and expression. For example, CNNs can identify transcription factor binding sites—regions of DNA where proteins interact to control gene activity.
This ability to “see” patterns in data makes CNNs perfect for tasks like classifying mutations or predicting the functional impact of genetic variations.
Recurrent Neural Networks (RNNs)
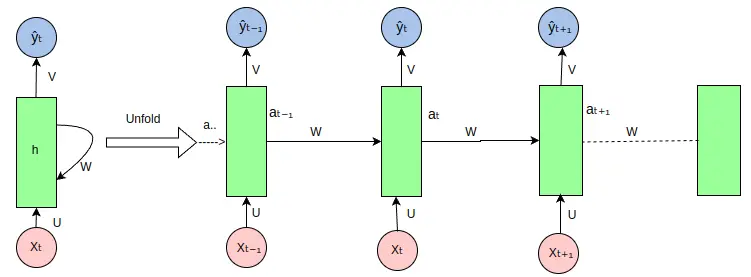
RNNs are designed to process sequential data, which makes them ideal for analyzing time-series genomic information, such as gene expression over time. Unlike CNNs, RNNs have a “memory” that allows them to retain information from earlier parts of the sequence while analyzing the later parts.
In genomics, RNNs are used to predict how genes are expressed under different conditions or over time. They’re also critical for modeling RNA sequences, helping scientists better understand how genetic information is translated into functional proteins.
Graph Neural Networks (GNNs)
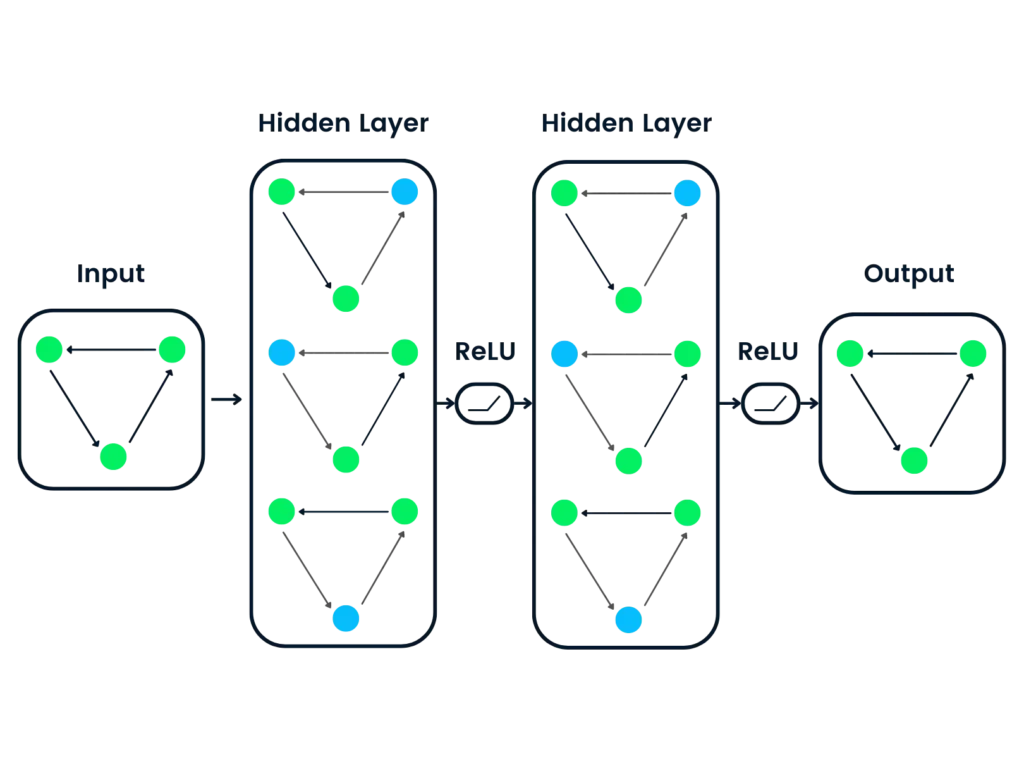
Genomic data is often represented as networks—such as gene regulatory networks or protein interaction networks—where nodes represent genes or proteins and edges represent their relationships. GNNs specialize in analyzing such data structures.
These networks help uncover the complex interactions between genes and proteins, leading to discoveries about how they work together to cause diseases or regulate biological processes. GNNs are particularly useful for studying pathways involved in cancer or neurological disorders.
Transformers
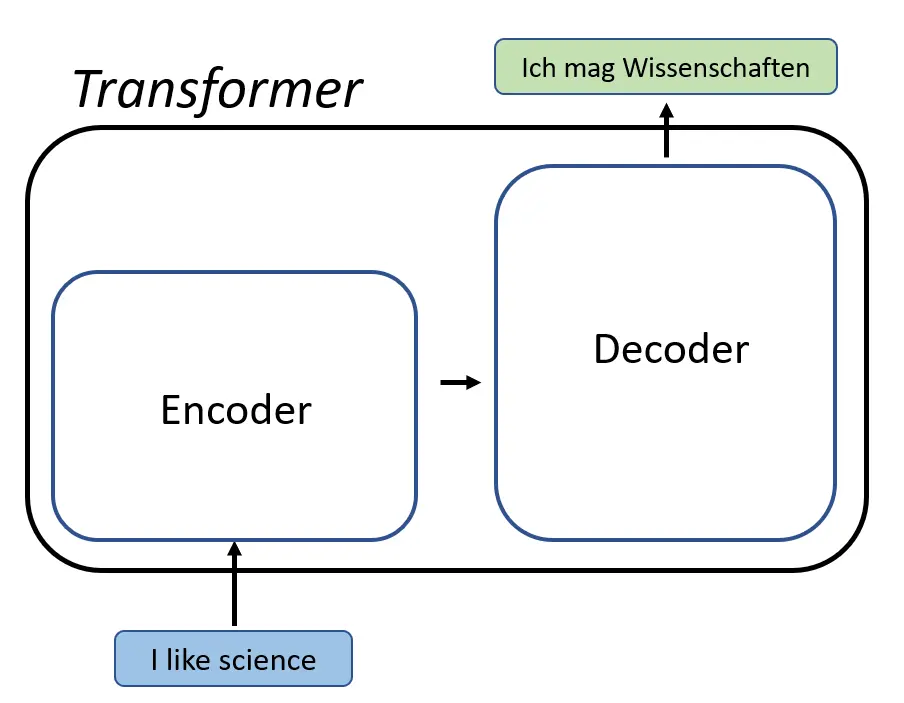
Transformers are among the most advanced deep learning architectures, known for their versatility and efficiency. Originally developed for natural language processing, transformers excel at analyzing long sequences, such as entire genes or large DNA regions. They process all parts of a sequence simultaneously, which makes them faster and more accurate than traditional RNNs for certain tasks.
In genomics, transformers are being used for tasks like variant calling, where they predict the likelihood that a specific genetic variation will impact health. Their ability to handle large-scale data makes them a powerful tool for genome-wide studies.
Read more: Transformers in Drug Design
Other Architectures
While CNNs, RNNs, GNNs, and transformers dominate the field, other architectures like autoencoders and generative adversarial networks (GANs) also contribute significantly.
- Autoencoders reduce the complexity of genomic data, making it easier to identify key features.
- GANs generate synthetic genomic data, which helps train models when real data is scarce.
Deep learning is transforming how we approach genomics, enabling us to uncover the secrets hidden within DNA and RNA. These models power many of the key applications you’ve already learned about, from precision medicine to drug discovery.
But how do we ensure these tools are used effectively? In the next section, we’ll explore Best Practices for Integrating AI in Genomic Projects, offering you actionable tips for success in this fast-evolving field. Let’s move forward with confidence!
Best Practices for Integrating AI in Genomic Projects
AI has enormous potential to advance genomic research, but success depends on how it is applied. Genomic data is complex, sensitive, and vast, so implementing AI requires thoughtful planning. The following best practices highlight the most important steps for integrating AI effectively into your genomic projects.
1. Use High-Quality and Clean Data
AI is only as good as the data it processes. Genomic data, being large and intricate, often contains errors, missing information, or inconsistencies that can mislead AI models. This is why data quality is the foundation of successful AI projects.
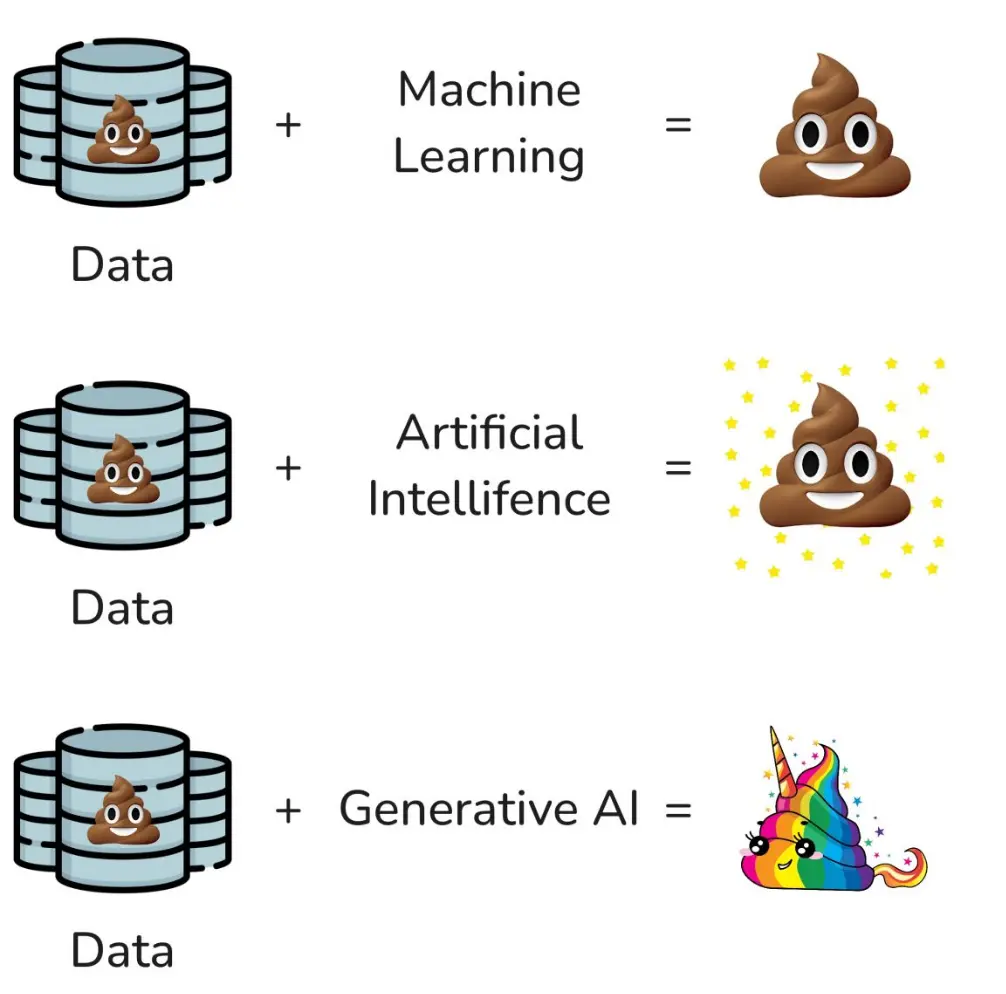
Start by rigorously checking your data for errors and anomalies. Remove any unreliable sequences and fill gaps with imputation methods designed to handle genomic datasets. Additionally, standardize your data formats to ensure consistency across experiments. By doing so, you set your AI models up for success, enabling them to produce accurate and meaningful results.
Without clean data, even the most advanced AI tools will fail to deliver reliable insights. Think of it like building a house: you need a solid foundation to support the entire structure.
2. Pick the Right AI Tools and Models
Choosing the right AI tools for your specific genomic task is critical. Different models excel at different types of analysis. For instance, convolutional neural networks (CNNs) are excellent for detecting patterns in DNA sequences, while recurrent neural networks (RNNs) are better suited for analyzing time-series data, such as changes in gene expression over time.
Graph neural networks (GNNs) are ideal for understanding relationships in gene networks, and transformers are powerful tools for handling large, complex sequences. However, selecting the right model can be challenging if you’re not familiar with these technologies.
This is where platforms like SilicoGene can guide you. Our intelligent platform analyzes your data and suggests the best models for your project, saving you time and improving results. Using the right tools ensures that your AI workflow is tailored to your specific research goals.
3. Make AI Models Easy to Understand
AI models can produce highly accurate results, but if their predictions are hard to explain, they may create uncertainty among your team or stakeholders. This is especially important in genomics, where decisions based on AI results can have significant consequences.
To address this, prioritize explainability. Choose AI models that provide insights into how they arrive at their predictions. Tools that highlight which data features influenced a decision can help you build trust in the results.
With the help of SilicoGene’s platform, you can easily implement explainable AI features. By doing so, you ensure that your team understands the outcomes and can confidently act on them. Transparency strengthens collaboration and prevents misunderstandings.
4. Protect Privacy and Follow the Rules
Genomic data is not just complex; it is also deeply personal and highly sensitive. Securing this data and complying with regulations, such as GDPR and HIPAA, is non-negotiable. Failing to do so can lead to severe legal, financial, and ethical consequences.
You must implement robust security measures, including encryption and strict access controls. Additionally, train your team to recognize and avoid common security risks. As the saying goes, “A chain is only as strong as its weakest link,” and you don’t want to be that weak link.
At SilicoGene, we emphasize shared responsibility for security. Our tools help you implement best practices and maintain compliance, ensuring that your data remains protected at all times.
5. Build a Strong Team
AI in genomics is a multidisciplinary effort. Successful projects require expertise in biology, data science, and ethics. Collaboration between these fields ensures that your project addresses all technical and ethical challenges.
Bring together biologists who understand the data’s biological relevance, data scientists who can build and refine AI models, and ethicists who can guide your project to adhere to ethical standards. Open communication and shared goals are key to success.
Using cloud platforms can further enhance collaboration by allowing your team to work together seamlessly, even across different locations. This not only improves efficiency but also fosters innovation through diverse perspectives.
6. Test and Validate AI Models Carefully
Testing is a crucial step in ensuring that your AI models perform well under real-world conditions. Even the most advanced model can fail if it hasn’t been properly validated.
Train your models on diverse datasets to confirm they can handle different scenarios. Use robust evaluation metrics, such as accuracy and recall, to measure performance. Pilot studies can help identify and fix issues before large-scale deployment.
At SilicoGene, we specialize in deploying AI models for health-tech applications. Our platform supports model validation and monitoring to ensure ongoing reliability. Serving and monitoring models is just as important as training them. Without proper oversight, issues like data drift can arise, reducing the effectiveness of your models over time. Monitoring ensures that your models remain accurate and ethical in the long term.
7. Plan for Growth
Genomic research often starts small but grows quickly as data accumulates and new questions emerge. To handle this growth, you need tools and systems that can scale with your needs.
Cloud computing platforms offer scalable resources, making it easier to store and analyze large datasets. Additionally, they support multi-omics integration, which combines data from genomics, proteomics, and more, giving you a complete picture of biological systems.
SilicoGene is designed to support scalability. Our platform manages everything from data preparation to model deployment, allowing you to focus on your research while we handle the technical details. By planning for growth, you future-proof your projects and ensure their long-term success.
By following these best practices, you can harness the full potential of AI in genomics while avoiding common pitfalls. In the next section, we’ll dive into real-world case studies that show how these strategies have been applied successfully. Let’s see what AI can achieve in action!
Real-World Case Studies Showcasing AI in Genomics
AI is already making a profound impact on genomics, with companies, universities, and research institutions using advanced AI tools to solve complex challenges. Let’s explore some real-world examples that highlight the power of AI in transforming genomic research and healthcare.
1. DeepMind’s AlphaFold: Revolutionizing Protein Structure Prediction
In 2024, DeepMind’s AlphaFold earned the Nobel Prize in Chemistry for its extraordinary contribution to protein structure prediction. This achievement resolved a challenge that had puzzled scientists for over 50 years. By accurately predicting the 3D structure of proteins from their amino acid sequences, AlphaFold brought a long-standing scientific dream to life. As a result, it has unlocked countless opportunities in biology and medicine.
Heiner Linke, Chair of the Nobel Committee for Chemistry, highlighted its significance, saying, “One of the discoveries being recognised this year is about fulfilling a 50-year-old dream: predicting protein structures from their amino acid sequences. Both of these discoveries open up vast possibilities.” This acknowledgment reflects the transformative impact of AlphaFold on the scientific community.
Since its release, AlphaFold2 has revolutionized research worldwide. More than two million researchers from 190 countries have used it, making it one of the most widely adopted AI tools in science. Moreover, its database now contains over 200 million protein structures, covering nearly all known organisms. This comprehensive resource has become invaluable to scientists working in diverse fields, including disease research, drug development, and evolutionary biology.
Institutions such as the European Molecular Biology Laboratory (EMBL) are leveraging AlphaFold to accelerate discoveries. For example, its ability to model protein structures has dramatically reduced the time needed to understand disease mechanisms and develop new therapies. Furthermore, its accessibility allows researchers to explore previously unexplored areas of structural biology.
By bridging the gap between theoretical predictions and practical applications, AlphaFold has set a new benchmark for computational biology. Its success demonstrates how AI, when applied thoughtfully, can address some of the most complex and critical challenges in science, paving the way for groundbreaking innovations in health and technology.
2. Tempus: AI-Driven Precision Medicine
Chicago-based healthcare company Tempus leverages AI to analyze genomic and clinical data to create personalized treatment plans for cancer patients. Their AI platform integrates data from tumor sequencing, clinical records, and imaging to help oncologists select the most effective therapies.
By combining genomic insights with AI-driven analytics, Tempus has improved cancer treatment outcomes and contributed to the development of targeted therapies.
3. University of California, San Francisco (UCSF): AI in Rare Disease Diagnosis
The UCSF Institute for Computational Health Sciences uses AI to identify genetic mutations responsible for rare diseases. Their approach combines machine learning and genome-wide association studies (GWAS) to uncover novel genetic variants linked to rare conditions.
This work has provided life-changing diagnoses for patients who previously lacked answers, demonstrating how AI can be a vital tool in precision medicine.
4. 23andMe: Consumer Genomics Powered by AI
Consumer genomics company 23andMe utilizes AI to analyze genetic data from millions of users. By applying machine learning to their vast dataset, 23andMe identifies genetic markers associated with diseases and traits.
Their research has contributed to the discovery of novel genetic variants linked to conditions like Parkinson’s disease and Type 2 diabetes. Furthermore, the company shares anonymized data with pharmaceutical companies to accelerate drug discovery.
5. Broad Institute and Microsoft: Cloud-Driven Genomic Analysis
The Broad Institute of MIT and Harvard partnered with Microsoft to leverage cloud computing and AI for large-scale genomic research. By integrating AI with scalable cloud platforms, the Broad Institute processes massive genomic datasets for projects like the Human Cell Atlas.
This partnership has improved the speed and efficiency of analyzing single-cell RNA sequencing data, leading to new insights into cell behavior and disease mechanisms.
6. Stanford University: AI for Gene-Editing Optimization
Stanford University researchers are using AI to improve the accuracy of CRISPR gene-editing technology. Their machine learning models predict off-target effects of CRISPR edits, allowing scientists to refine the technology and reduce unintended consequences.
This work is advancing gene-editing research, bringing it closer to clinical applications in treating genetic disorders.
7. Illumina and Grail: Early Cancer Detection with AI
Illumina, a leading genomics company, collaborated with Grail, a startup focused on early cancer detection, to develop AI-powered liquid biopsy tests. These tests analyze genomic material in the bloodstream to detect cancers at their earliest stages.
Using AI to identify subtle patterns in genomic data, Grail’s technology has demonstrated high sensitivity in detecting multiple types of cancer, even before symptoms appear. This has the potential to save countless lives through early intervention.
8. Oxford Nanopore Technologies: Real-Time Sequencing with AI
UK-based Oxford Nanopore Technologies uses AI to enhance real-time DNA and RNA sequencing. Their platform employs neural networks to analyze sequencing data and detect genetic variations, including those relevant to infectious diseases and rare conditions.
Their AI-driven technology has been instrumental in sequencing pathogens during outbreaks, such as identifying COVID-19 variants in record time.
These case studies demonstrate the transformative potential of AI in genomics, from improving precision medicine to enabling groundbreaking discoveries in biology. As more organizations adopt AI, the possibilities for innovation will only continue to grow.
The Future of AI in Genomics
The future of genomics is deeply intertwined with the evolution of artificial intelligence (AI). With rapid advancements in AI technology and the continuous growth of genomic data, we are on the brink of a revolution that will reshape medicine, biology, and our understanding of life itself. Let’s explore some key trends and predictions supported by current evidence.
1. Multi-Omics Integration for Holistic Insights
Genomics is no longer an isolated field. The integration of genomics with other omics data—such as transcriptomics, proteomics, and metabolomics—provides a more comprehensive understanding of biological systems. AI is already enabling multi-omics integration by analyzing these diverse datasets together to uncover connections that were previously hidden.
For example, recent studies have shown how deep learning models can link gene expression patterns to protein interactions and metabolic pathways. These insights are crucial for developing targeted therapies and personalized medicine. As AI becomes more powerful, we can expect even greater breakthroughs in understanding complex diseases, such as cancer and neurodegenerative disorders.
2. Real-Time Genomic Analysis with AI and Cloud Computing
The combination of AI and cloud computing is making real-time genomic analysis a reality. Platforms like Google Cloud and AWS are already being used to analyze large-scale genomic data quickly and efficiently. This trend is particularly important for applications such as infectious disease monitoring and outbreak response.
For instance, during the COVID-19 pandemic, AI-driven genomic analysis was instrumental in tracking virus mutations. In the future, this capability will expand to other pathogens, enabling us to respond faster to emerging health threats and preventing global outbreaks.
3. Quantum Computing and AI: The Next Frontier
While still in its infancy, quantum computing has the potential to revolutionize genomic research by solving problems that are computationally infeasible for classical computers. AI models used in genomics require significant computational power, especially for tasks like genome-wide association studies (GWAS) or protein structure predictions. Quantum computing, when combined with AI, could accelerate these processes exponentially.
Researchers are already exploring how quantum algorithms can optimize AI models for genomic data. As this technology matures, it will likely open up new possibilities, from faster drug discovery to the analysis of ultra-complex genetic datasets.
4. Advances in Explainable and Ethical AI
The future of AI in genomics will prioritize transparency and ethics. Explainable AI (XAI) will become a standard practice, ensuring that models provide clear and interpretable results. This is especially critical in healthcare, where decisions must be evidence-based and understandable to clinicians and patients alike.
Additionally, there will be a stronger emphasis on fairness and bias reduction. AI systems will need to account for the genetic diversity of global populations, which has been a significant challenge in current genomic research. By developing inclusive datasets and validating AI models across diverse populations, the field will ensure equitable benefits for all.
5. Accelerating Personalized Medicine
Personalized medicine is one of the most promising applications of AI in genomics. As AI models improve, they will enable faster and more accurate identification of genetic markers associated with diseases. This, in turn, will lead to better-tailored treatments based on an individual’s unique genetic makeup.
For example, Tempus and 23andMe have already demonstrated the power of AI in providing actionable insights for cancer and genetic risk assessment. Moving forward, AI will integrate lifestyle and environmental factors with genetic data, creating even more precise recommendations for disease prevention and management.
6. Democratization of Genomic Research
AI-powered tools are making genomic research more accessible to smaller institutions and researchers in developing countries. Platforms like AlphaFold2 and SilicoGene have lowered the barriers to entry by providing user-friendly tools for analyzing genomic data. This democratization will expand participation in genomics, leading to more diverse contributions and faster innovation.
7. The Expansion of AI in Gene Editing
AI will continue to enhance gene-editing technologies like CRISPR. Machine learning models are already being used to predict off-target effects, improving the accuracy and safety of gene edits. In the future, AI could design entirely new gene-editing tools tailored to specific genomes or applications, paving the way for more precise genetic therapies.
The combination of these advances and emerging technologies like quantum computing ensures that the future of AI in genomics is incredibly bright. Researchers, companies, and institutions are poised to drive transformative discoveries that will redefine healthcare and unlock the full potential of the human genome.
The journey has just begun, and the possibilities are endless.
Frequently Asked Questions (FAQs) About Applications AI in Genomics
1. How is AI used in genomics?
AI is used in genomics to analyze large and complex genetic datasets, uncover patterns, and make predictions that are difficult or impossible for traditional methods. For example, AI can identify genetic variants linked to diseases, predict how specific genes are expressed under different conditions, and assist in drug discovery. Machine learning and deep learning models, such as CNNs and transformers, play a significant role in processing genomic sequences and extracting meaningful insights.
2. Can AI predict genetic diseases?
Yes, AI can predict genetic diseases by analyzing DNA sequences, family history, and other factors. Predictive models use machine learning to identify patterns in genetic data that indicate a predisposition to conditions like cancer, diabetes, or rare genetic disorders. These predictions help doctors and researchers take preventative measures or develop personalized treatment plans, improving patient outcomes.
3. What are the ethical concerns associated with AI in genomics?
Ethical concerns in AI-driven genomics primarily involve privacy, bias, and data ownership. Genomic data is highly sensitive and personal, so protecting it from unauthorized access is crucial. Additionally, AI models can sometimes inherit biases from the datasets they are trained on, leading to unequal outcomes. Finally, questions around who owns and controls genomic data raise important legal and ethical considerations. Ensuring compliance with regulations like GDPR and HIPAA and fostering transparency in AI systems are vital steps in addressing these challenges.
4. What is explainable AI, and why is it important in genomics?
Explainable AI refers to models and techniques that make it clear how and why an AI system produces certain results. In genomics, this is especially important because decisions based on AI outputs can have significant health implications. For instance, an explainable model can show which genetic factors contributed to a disease prediction, helping researchers validate findings and clinicians trust recommendations. This transparency is crucial for building confidence in AI systems and ensuring they are used responsibly.
5. How does AI accelerate drug discovery in genomics?
AI accelerates drug discovery by analyzing genetic data to identify potential drug targets and predict how new compounds will interact with specific genes or proteins. This significantly reduces the time and cost associated with traditional drug development methods. For example, AI can rapidly screen millions of molecules to find those most likely to work against a particular disease. Companies like Tempus and Illumina are leveraging AI to develop treatments faster and more efficiently, demonstrating its potential to revolutionize the pharmaceutical industry.
Conclusion
AI is transforming genomics, unlocking opportunities that were once unimaginable. From predicting protein structures with tools like AlphaFold to enabling precision medicine and accelerating drug discovery, AI is reshaping how we understand and apply genetic information. These advancements, supported by explainable and ethical AI practices, are not only pushing the boundaries of science but also ensuring that the benefits of genomics are accessible, equitable, and impactful.
As we look ahead, the integration of emerging technologies like quantum computing and multi-omics analysis promises even greater breakthroughs. However, these innovations come with challenges that demand careful planning, ethical consideration, and collaboration across disciplines.
How will these advancements redefine the boundaries of healthcare and biology? And what new possibilities might AI unlock in the study of life itself? The answers lie in the exciting journey ahead—one where AI continues to unravel the complexities of the genome and, in doing so, transforms the future of medicine and research.