What if we could solve humanity’s most pressing biological challenges—faster and with greater precision? That’s the promise of artificial intelligence (AI) in biology. By decoding massive datasets, AI is revolutionizing drug discovery, tailoring treatments to individuals, optimizing agriculture, and even protecting the planet.
This article dives deep into the transformative applications of AI in biology. From groundbreaking research to real-world solutions, here’s everything you need to know about how AI is reshaping life sciences and why it matters to you.
What is AI in Biology?
At its core, AI mimics human intelligence but operates at a scale and speed we can’t match. In biology, AI processes complex datasets—from genetic codes to environmental signals—turning them into actionable insights.
For example, AI-powered tools like DeepMind’s AlphaFold have solved decades-old problems like predicting protein structures, paving the way for advances in drug development and synthetic biology. AI also underpins tools like IBM Watson, which helps oncologists devise personalized cancer treatments.
Think of AI as a translator for biology, transforming chaos into clarity and helping us unlock nature’s secrets faster than ever.
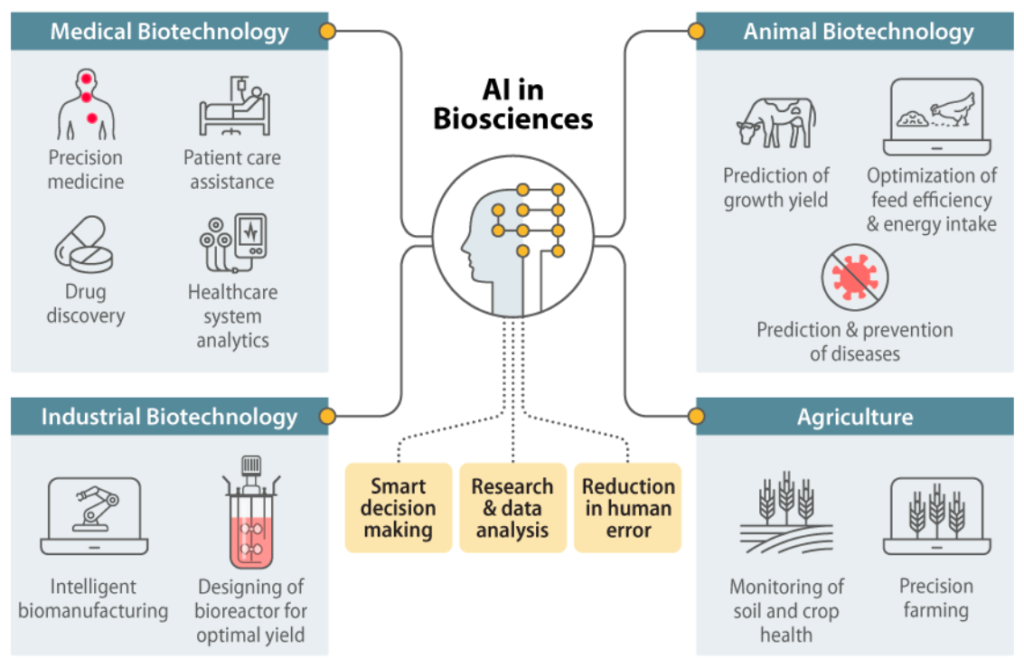
How AI is Revolutionizing Biology
Discovery and Development
The process of drug discovery and development has long been synonymous with time, expense, and uncertainty. Traditionally, it takes over a decade and billions of dollars to bring a single drug to market. Researchers have to identify drug targets, screen thousands of compounds, and navigate rigorous preclinical and clinical trials. Along the way, failure rates remain dauntingly high, with over 90% of candidates never reaching approval. This inefficiency leaves many diseases without effective treatments and contributes to rising healthcare costs.
Artificial intelligence is rewriting this narrative by making the process faster, more precise, and less costly. AI accelerates target identification by analyzing vast datasets, including scientific literature, clinical trial results, and genetic databases. For example, Insilico Medicine demonstrated this potential by identifying a drug candidate for fibrosis in just 46 days—an achievement that would typically take several years. Additionally, AI tools like those from Atomwise use molecular simulations to predict how small molecules bind to proteins, significantly enhancing precision in early-stage research.
AI’s role doesn’t stop at discovery; it extends into risk reduction during clinical trials. By analyzing patient data, including demographics and genetic markers, AI can predict how drugs will perform in specific populations, avoiding costly failures. These advancements mean that life-saving treatments can reach patients faster and at a fraction of the traditional cost.
Why AI Matters in Discovery and Development:
- Time Savings: AI reduces the timeline for identifying viable drug candidates, enabling faster responses to emerging health crises.
- Cost Efficiency: Streamlined research processes significantly lower the financial barriers to drug development.
- Improved Precision: AI-driven simulations predict drug interactions and outcomes more accurately than traditional methods.
With AI’s transformative capabilities, the pharmaceutical industry is poised to address unmet medical needs while redefining how we approach innovation in healthcare.
Personalized Medicine
For decades, healthcare has operated on a one-size-fits-all model. However, diseases like cancer and diabetes rarely behave uniformly across individuals. What works for one person may fail for another due to unique genetic, environmental, and lifestyle factors. Personalized medicine addresses this disparity by tailoring treatments to each patient’s biology, offering a level of care that was previously unattainable.
Personalized medicine is especially crucial for conditions like cancer, where treatments often involve significant trial and error. Consider a patient undergoing chemotherapy only to discover, after months of treatment, that their tumor didn’t respond. Such inefficiencies not only waste time but also put patients at unnecessary risk. This is where AI becomes a game-changer, providing actionable insights that drive precision and efficacy.
AI systems analyze massive amounts of genetic and clinical data to uncover patterns and correlations that humans might miss. For instance, IBM Watson aids oncologists in developing personalized cancer therapies by identifying specific genetic mutations and recommending targeted treatments. Similarly, Tempus applies machine learning to integrate clinical and molecular data, creating individualized treatment plans that improve outcomes for complex diseases.
How AI is Revolutionizing Personalized Medicine:
- Data Analysis: AI identifies genetic markers linked to disease susceptibility, enabling preventive care and early intervention.
- Targeted Treatments: By predicting drug efficacy for specific mutations, AI reduces adverse reactions and increases treatment success rates.
- Comprehensive Care Plans: AI integrates diverse datasets to provide doctors with holistic, patient-specific recommendations.
As AI continues to advance, personalized medicine is shifting from being a luxury to a standard of care. With treatments optimized for individual biology, patients can expect better outcomes, fewer side effects, and a healthcare system that truly puts their needs first.
Agricultural Innovations
Agriculture faces unprecedented challenges. Feeding a global population expected to exceed 9 billion by 2050 requires increasing food production while battling climate change, resource scarcity, and biodiversity loss. Traditional farming methods, though effective in the past, are no longer sustainable in the face of these mounting pressures. This is where AI steps in to transform the industry.
By leveraging machine learning and data analysis, AI optimizes farming practices to improve yields and reduce waste. For instance, AI-powered drones now monitor crops, providing real-time data on plant health and detecting early signs of disease. This timely intervention prevents the spread of infections that could devastate entire harvests. Moreover, systems like John Deere’s precision agriculture tools identify weeds and apply herbicides only where needed, cutting chemical use significantly.
AI’s impact extends beyond crop management. Smart irrigation systems analyze environmental data to determine the exact amount of water needed, conserving a vital resource while ensuring healthy crops. In addition, platforms such as PEAT use AI-driven image recognition to diagnose plant diseases, allowing farmers to respond quickly and avoid losses.
Why AI is Vital in Agriculture:
- Sustainability: AI reduces waste by optimizing water, fertilizer, and pesticide use.
- Disease Control: Early detection tools prevent widespread crop damage.
- Increased Productivity: Precision farming techniques maximize yields while minimizing costs.
As we look to the future, AI’s ability to balance productivity with sustainability makes it a cornerstone of modern agriculture. By addressing today’s challenges, it paves the way for a food-secure tomorrow.
Image Processing and Diagnostics
Medical diagnostics are at the heart of effective healthcare, yet they remain fraught with challenges. Diseases like cancer often go undetected in their early stages due to human error or a lack of resources. Moreover, the growing demand for diagnostic services has put immense pressure on radiologists and pathologists worldwide. AI offers a solution by improving both the accuracy and efficiency of medical image analysis.
AI excels at identifying patterns in complex medical images, detecting anomalies that even skilled professionals might overlook. For example, Google’s DeepMind developed a model capable of diagnosing over 50 retinal diseases with accuracy comparable to expert ophthalmologists. Similarly, PathAI’s technology analyzes pathology slides to identify malignant tissues with remarkable precision.
Beyond accuracy, AI enhances speed. While a radiologist might take hours to analyze multiple images, an AI system can process thousands in minutes, providing results that guide immediate clinical decisions. These advancements are particularly impactful in underserved regions, where access to specialists is limited.
How AI Transforms Diagnostics:
- Early Detection: AI identifies diseases like cancer and diabetes at earlier stages, improving outcomes.
- Enhanced Precision: Algorithms minimize diagnostic errors, leading to more reliable results.
- Global Accessibility: AI-powered tools bring advanced diagnostics to regions lacking medical infrastructure.
With AI, the future of diagnostics is not just faster and more accurate—it’s accessible to everyone, regardless of geography or resources.
Data Integration and Knowledge Discovery
Biological research generates an overwhelming amount of data, from genomic sequences to clinical trial results. Yet, much of this information remains siloed in disparate systems, making it difficult for researchers to draw meaningful connections. The challenge is not just managing the volume of data but also synthesizing it into actionable insights. Here, AI proves indispensable.
AI integrates diverse datasets, revealing patterns and correlations that humans might miss. Tools like BenevolentAI analyze vast collections of biomedical literature to identify potential drug targets, accelerating the discovery process. Similarly, SciBite streamlines literature reviews, helping researchers navigate complex datasets quickly and effectively. These capabilities allow scientists to focus on innovation rather than spending countless hours sifting through raw data.
Moreover, AI’s ability to recognize patterns enables groundbreaking discoveries. For example, by analyzing clinical data alongside genomic studies, AI has uncovered links between genetic mutations and rare diseases. This not only improves our understanding of disease mechanisms but also informs the development of targeted therapies.
Key Benefits of AI in Data Integration:
- Efficiency: AI consolidates fragmented data, saving researchers time and resources.
- Discovery: Pattern recognition tools uncover hidden relationships between datasets.
- Scalability: AI processes data at a scale impossible for humans, opening new frontiers in research.
As the volume of biological data continues to grow, AI’s role in integration and discovery will only become more crucial. It is not just helping us make sense of the present—it is unlocking the future of science.
Challenges in AI for Biology
Artificial intelligence is transforming biology, but it faces significant challenges that require attention and resolution. These hurdles, while surmountable, can limit AI’s potential if not addressed. Here are some of the key challenges:
- Data Quality and Accessibility
Biological datasets are often incomplete, fragmented, or stored across unconnected systems. Poor data quality reduces the effectiveness of AI models, making accurate predictions difficult. Efforts like the Human Cell Atlas are helping by creating unified, high-quality datasets, but more collaboration and standardization are needed. - Ethical Concerns
The use of AI in sensitive areas, such as genetic editing or healthcare decision-making, raises critical ethical questions. Issues around privacy, informed consent, and potential misuse of technologies like CRISPR must be addressed to ensure AI benefits everyone fairly. Discussions are already underway to create ethical frameworks, but their implementation requires global cooperation. - Algorithmic Bias
AI models are only as unbiased as the data they are trained on. If datasets lack diversity, the resulting algorithms may disproportionately benefit or harm specific groups. This bias can lead to unequal healthcare outcomes or flawed research findings, emphasizing the need for inclusive data practices. - Interdisciplinary Collaboration
Successful AI applications require teamwork between biologists, data scientists, and engineers. However, these disciplines often operate in silos, slowing progress. Institutions like the Chan Zuckerberg Biohub are fostering collaboration by uniting experts from different fields, but broader participation is necessary.
By addressing these challenges, we can pave the way for more reliable, ethical, and impactful applications of AI in biology.
Future Directions for AI in Biology
The future of AI in biology is filled with groundbreaking possibilities. As technology evolves, it will open doors to new applications and deeper scientific understanding. These advancements will drive innovation across multiple fields:
- AI-Driven Autonomous Laboratories
Imagine a lab where experiments run themselves. Fully autonomous labs, like those at the Rosalind Franklin Institute, are becoming a reality. These labs use AI to design, conduct, and analyze experiments with minimal human input. This efficiency accelerates discoveries, reduces errors, and frees researchers to focus on creative problem-solving. - Advanced Predictive Models
Predictive models powered by AI will become even more sophisticated. Tools like DeepMind’s AlphaFold, which predicts protein structures, have already revolutionized molecular biology. Future models could simulate enzyme reactions or predict metabolic pathways, enabling breakthroughs in synthetic biology and drug design. - Applications in Bioengineering
AI is set to play a central role in designing new organisms and biomaterials. Companies like Ginkgo Bioworks are leveraging AI to engineer microorganisms with specific traits. These innovations could lead to sustainable biofuels, advanced pharmaceuticals, and resilient crops for agriculture. - Integration with Multi-Omics Data
Combining genomics, proteomics, and metabolomics through AI will deepen our understanding of complex biological systems. This integration allows researchers to analyze life at multiple levels, providing insights that were previously unattainable. - Global Accessibility
AI tools are making advanced biology more accessible worldwide. With cloud-based platforms and affordable diagnostic tools, researchers and healthcare providers in underserved regions can benefit from cutting-edge technology.
These advancements promise a future where AI isn’t just a tool—it’s a partner in scientific discovery, pushing the boundaries of what we know and how we apply that knowledge to improve lives.
FAQs
Q1: What is AI in biology?
AI in biology refers to the application of artificial intelligence technologies, such as machine learning and data analysis, to solve complex biological problems. This includes tasks like analyzing genetic data, predicting protein structures, and discovering new drugs.
Q2: Why is AI important in biology?
AI is crucial because it processes vast amounts of biological data far faster and more accurately than humans. This capability accelerates research, reduces costs, and enables innovations in medicine, agriculture, and environmental science.
Q3: How is AI used in biological research?
AI is used to identify patterns in genetic sequences, predict disease outcomes, automate laboratory experiments, and even design synthetic organisms. It’s a tool that enhances the precision and efficiency of biological studies.
Q4: How does AI accelerate drug discovery?
AI analyzes large datasets from clinical trials, scientific publications, and molecular databases to identify promising drug candidates. It also predicts how drugs will interact with biological targets, shortening the development timeline from years to months.
Q5: What is personalized medicine, and how does AI contribute to it?
Personalized medicine tailors treatments to an individual’s unique genetic makeup and clinical data. AI helps by analyzing this information to predict how a patient will respond to specific treatments, ensuring better outcomes with fewer side effects.
Q6: Can AI completely replace human researchers in drug discovery?
No. While AI automates data analysis and identifies potential drug targets, human expertise is still essential for interpreting results, designing experiments, and ensuring ethical considerations are met.
Q7: What are the biggest challenges of using AI in biology?
Key challenges include poor data quality, lack of standardization, and ethical concerns related to privacy and algorithmic bias. Addressing these issues requires collaboration across disciplines and the development of clear regulatory frameworks.
Conclusion: Transforming Biology with AI
Artificial intelligence is not just a tool—it’s a revolution driving biology toward unprecedented possibilities. From accelerating drug discovery to tailoring personalized medicine, optimizing agriculture, enhancing diagnostics, and integrating complex datasets, AI is reshaping the way we understand and improve life. Its ability to tackle challenges that were once insurmountable is opening new doors in healthcare, agriculture, and environmental sustainability.
As we move forward, the future of AI in biology is brighter than ever. With advancements in autonomous laboratories, predictive modeling, and bioengineering, the potential for innovation is limitless. However, realizing this vision requires collaboration, ethical governance, and a commitment to pushing boundaries.
Ready to unlock the power of AI for your biology project? Whether you’re looking to innovate in drug discovery, optimize agricultural systems, or solve a unique biological challenge, we’re here to help. Let’s work together to bring your ideas to life with cutting-edge AI solutions.
References:
- Bhardwaj, A., Kishore, S., & Pandey, D. K. (2022). Artificial Intelligence in Biological Sciences. Life (Basel), 12(9), 1430. https://doi.org/10.3390/life12091430. PMID: 36143468; PMCID: PMC9505413.
- Hassoun, S., Jefferson, F., Shi, X., Stucky, B., Wang, J., & Rosa, E. (2021). Artificial Intelligence for Biology. Integrative and Comparative Biology, 61(6), 2267–2275. https://doi.org/10.1093/icb/icab188.
- Fink, O., Hartung, T., Lee, S. Y., & Maynard, A. (2024). AI for scientific discovery: Pioneering new frontiers in knowledge. In Top 10 Emerging Technologies of 2024. World Economic Forum. Published June 25, 2024.
- Holzinger, A., Keiblinger, K., Holub, P., Zatloukal, K., & Müller, H. (2023). AI for life: Trends in artificial intelligence for biotechnology. New Biotechnology, 74, 16–24. https://doi.org/10.1016/j.nbt.2023.02.001