What if we could design life-saving drugs faster, cheaper, and more effectively than ever before? AI in drug design is making this dream a reality, transforming a traditionally time-consuming and expensive process into an efficient, data-driven innovation hub. With costs exceeding $2.8 billion and over a decade required to develop a single drug, the pharmaceutical industry faces immense pressure to innovate. Enter artificial intelligence: an approach that accelerates molecule discovery, optimizes drug properties, and opens vast chemical spaces previously beyond human reach.
In this article, we’ll explore the groundbreaking role of AI in drug design, focusing on key applications like de novo drug generation, molecule simulations, virtual screening, and ADMET predictions. Whether you’re a researcher, entrepreneur, or enthusiast, this guide offers actionable insights into how AI is reshaping medicine.
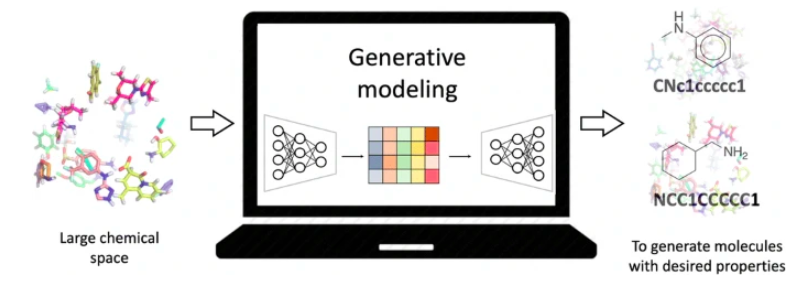
Understanding AI in Drug Design
What is AI in Drug Design?
Artificial intelligence in drug design refers to the application of machine learning (ML) and deep learning (DL) technologies to identify, optimize, and develop new therapeutic compounds. Unlike traditional methods that rely on exhaustive trial-and-error or heuristic-driven experimentation, AI leverages vast datasets, advanced algorithms, and computational power to streamline the process. This approach allows researchers to model complex biological interactions, predict drug efficacy, and even create novel molecular structures from scratch.
At its core, AI in drug design integrates techniques like:
- Deep Generative Models (DGMs): For creating entirely new molecules based on desired properties.
- Reinforcement Learning: To iteratively refine molecules toward optimal biological activity.
- Natural Language Processing (NLP): To analyze and extract insights from research data or chemical literature.
Why Generative AI is a Game-Changer
Generative AI has transformed the way we think about drug discovery. Unlike traditional methods confined to existing chemical libraries, generative AI explores vast chemical spaces, generating novel compounds tailored to specific biological targets. This innovation has already proven effective, with success stories like inhibitors designed in just 21 days using generative adversarial networks (GANs).
Key benefits include:
- Efficiency: Reduces time from discovery to preclinical trials by automating key steps.
- Precision: Enhances accuracy in predicting how molecules will interact with biological targets.
- Cost-Effectiveness: Saves millions by identifying promising candidates early in the pipeline.
Key Applications of AI in Drug Design
The role of AI in drug design extends far beyond accelerating the process—it’s reshaping how we create, test, and refine therapeutic compounds. By tackling complex problems like creating entirely new molecules, predicting drug safety, and simulating molecular behavior, AI provides a powerful toolkit for modern drug discovery. Here’s how AI is applied across key stages of the process.
De Novo Drug Design: Crafting Molecules from Scratch
Imagine being able to create a new drug molecule tailored specifically to treat a disease—something that’s never existed before. That’s the power of de novo drug design, where AI models generate novel compounds from scratch. These models use mathematical representations of chemical structures to explore vast chemical spaces that are impossible for humans to navigate.
How It Works:
- AI models like Generative Adversarial Networks (GANs) and Variational Autoencoders (VAEs) take molecular data and learn patterns to generate new molecules with specific properties.
- These molecules are evaluated for key attributes like structural stability, biological activity, and chemical feasibility.
Metrics for Success:
- Novelty: How different is the generated molecule from existing drugs? AI models can produce unique structures while maintaining “drug-like” properties.
- Drug-Likeness Scores: Metrics like QED (Quantitative Estimate of Drug-likeness) measure how suitable a molecule is for therapeutic use.
- Success Rates: AI models have been shown to identify viable drug candidates in weeks, a process that previously took years.
For instance, AI-generated molecules are now being tested for their ability to target diseases with high precision. These designs not only accelerate discovery but also allow researchers to think outside the constraints of existing chemical libraries.
Drug Optimization: Fine-Tuning Molecules for Better Results
Designing a molecule is just the beginning—it often needs refinement to meet clinical requirements. AI helps improve known molecules by modifying their structure to enhance properties like potency, stability, or safety.
Examples of Optimizations:
- Increasing Potency: AI can predict structural changes that make a drug bind more strongly to its target.
- Reducing Side Effects: By analyzing toxicity data, AI identifies elements in the molecule that could cause harmful reactions and suggests alternatives.
- Enhancing Selectivity: AI ensures the drug interacts with the intended biological target while avoiding others, minimizing unintended effects.
Why This Matters: In traditional workflows, these adjustments require trial-and-error experimentation in labs—a time-consuming and costly process. AI accelerates this by providing precise recommendations based on large datasets of known interactions.
Molecule Simulations and In Silico Testing
Testing how a drug will interact with biological systems is critical, but it’s also one of the most resource-intensive steps. AI streamlines this through in silico testing—virtual experiments that simulate molecular behavior without the need for physical trials.
What AI Simulations Achieve:
- Protein-Ligand Binding Studies: AI predicts how strongly a drug binds to its target protein, a key measure of effectiveness.
- Molecular Dynamics: Simulations reveal how a molecule behaves in a dynamic environment, such as within a human cell.
- Geometry and Stability Analysis: AI evaluates the three-dimensional structure of molecules to ensure they remain stable and functional.
Metrics Used:
- Binding Affinity Scores: These indicate how effectively a drug molecule attaches to its target, with higher scores suggesting stronger interactions.
- Pose Accuracy: Measures how closely a simulated binding interaction aligns with expected outcomes.
By running thousands of simulations in a fraction of the time, AI reduces the need for expensive laboratory tests and ensures only the most promising candidates proceed to the next stage.
Virtual Screening and Expanding Compound Libraries
AI makes it possible to search through vast libraries of chemical compounds to identify those with the highest likelihood of success. Virtual screening, powered by AI, evaluates millions of molecules in days rather than months, ranking them based on how well they meet predefined criteria.
How AI Enhances Virtual Screening:
- Speed: Traditional screening methods are slow, but AI can analyze thousands of molecules per hour.
- Prioritization: AI ranks compounds based on their predicted effectiveness, focusing attention on the most promising candidates.
- Library Expansion: AI also generates new compounds by combining features of existing ones, further broadening the search space.
Benefits for Researchers: Instead of blindly testing large libraries, researchers can target the top-performing molecules identified by AI. This focused approach not only saves time but also dramatically cuts costs.
Predicting ADMET Properties: Ensuring Safety and Efficacy
A drug isn’t just about killing harmful cells—it must also be safe for the rest of the body. AI helps predict a molecule’s Absorption, Distribution, Metabolism, Excretion, and Toxicity (ADMET) properties long before clinical trials begin.
Why ADMET Prediction Matters:
- Avoiding Failures: Many drug candidates fail in late-stage trials due to unforeseen safety issues. AI addresses this by identifying risks early.
- Optimizing Delivery: AI predicts how well a drug will be absorbed into the bloodstream and reach the target site.
- Minimizing Side Effects: Toxicity predictions highlight potential dangers, allowing chemists to modify molecules proactively.
What AI Evaluates:
- Bioavailability: How easily the drug enters the bloodstream.
- Toxicity Levels: Identifies risks like liver damage or harmful interactions with other drugs.
- Clearance Rates: Determines how quickly the body can process and eliminate the drug.
By providing detailed insights into these critical factors, AI reduces costly trial-and-error experiments and increases the likelihood of success.
Through applications like these, AI isn’t just speeding up drug design—it’s revolutionizing how we approach it. These technologies bring precision, scalability, and efficiency to every stage, from creating molecules to ensuring they are safe and effective for human use. The result? Faster, more cost-effective pathways to life-saving treatments.
Challenges and Opportunities in AI-Driven Drug Design
The integration of AI in drug design has brought tremendous advancements, but it is not without challenges. As researchers push the boundaries of what’s possible, they face hurdles in data quality, regulatory acceptance, and the inherent complexities of biological systems. At the same time, these challenges pave the way for innovation, creating opportunities to refine AI applications and extend their impact.
Data Challenges and the Quest for Quality
AI models depend on high-quality data to deliver accurate predictions, but the pharmaceutical field often grapples with incomplete or biased datasets. For example, many datasets used for training AI lack diversity in chemical and biological representation, making it difficult to generalize findings across various diseases or target proteins.
Adding to this is the scarcity of 3D structural data for molecules, which limits the effectiveness of models that rely on spatial information. While 2D representations like SMILES strings are widely used, they fail to capture the full complexity of molecular behavior.
Opportunity: Advances in data augmentation and the development of synthetic datasets are helping to overcome these limitations. For instance, AI can now generate diverse chemical structures that enrich training datasets, making models more robust and generalizable.
Model Generalization and Scalability
Another challenge is creating AI models that perform well across diverse targets and chemical spaces. Many existing models are highly specialized, excelling with specific datasets but struggling when applied to new or unseen problems. This lack of generalization can result in overfitting and reduced reliability.
Generative AI models are also computationally intensive, requiring significant resources to train and validate. The high cost of implementation, both in terms of time and infrastructure, remains a barrier for smaller research teams or startups.
Opportunity: Modular AI frameworks are being developed to adapt to new tasks with minimal retraining. Techniques like transfer learning allow models trained on one type of data, such as small molecules, to be fine-tuned for another, like protein-ligand interactions, reducing resource demands.
Balancing Innovation with Regulation
One of the most significant challenges in AI-driven drug design is navigating regulatory frameworks. Drug candidates must not only demonstrate efficacy but also meet stringent safety standards. AI models, often perceived as “black boxes,” can lack the transparency needed for regulatory approval.
For example, regulators may question how an AI-generated molecule was designed or why it is expected to succeed. Without clear, explainable outputs, gaining approval becomes an uphill battle.
Opportunity: Explainable AI (XAI) techniques are making AI models more interpretable. By providing insights into how a molecule was designed and the reasoning behind its predicted efficacy, these methods enhance trust and facilitate smoother regulatory pathways.
The Future of Generative AI in Drug Design
As the field of AI in drug design evolves, it is poised to become even more transformative. From personalized medicine to industry-wide collaboration, the possibilities for generative AI are vast.
The Rise of Personalized Medicine
Imagine a world where medications are tailored specifically to your genetic profile. Generative AI is making this a reality by analyzing genetic, clinical, and lifestyle data to design drugs that work best for individual patients. This approach not only improves efficacy but also reduces adverse effects.
One promising application is in oncology, where AI designs treatments targeting specific mutations in cancer cells. By personalizing therapies, patients receive drugs that are more effective against their unique cancer profile, potentially improving survival rates and quality of life.
Collaborative Innovation Across Industries
The future of AI-driven drug design will likely be shaped by collaboration. Partnerships between pharmaceutical companies, AI startups, and academic institutions are already driving breakthroughs. By pooling expertise, these collaborations are accelerating the development of innovative solutions.
For example, pharmaceutical companies provide access to proprietary datasets, while AI firms contribute cutting-edge algorithms. Academic researchers often serve as a bridge, offering foundational insights that tie these efforts together. This ecosystem not only speeds up drug discovery but also democratizes access to advanced technologies.
Expanding Chemical Exploration
Generative AI’s ability to explore vast chemical spaces remains one of its most exciting prospects. Future models will integrate even more data types—such as real-world patient outcomes and environmental factors—to refine their predictions. This expansion will help researchers identify molecules with not only therapeutic potential but also sustainability in production.
One emerging trend is the incorporation of 3D-aware generative models, which are specifically designed to understand spatial relationships in molecular structures. These models will likely set a new standard in designing drugs that can bind precisely to their targets with minimal off-target effects.
FAQs: Common Questions About AI in Drug Design
- What is AI in drug design?
AI in drug design refers to the use of artificial intelligence technologies to accelerate and optimize the discovery, development, and testing of new drugs. By leveraging machine learning models and vast datasets, AI can predict molecular interactions, generate novel compounds, and refine drug properties with unparalleled efficiency.
- How does AI generate new drug molecules?
AI uses generative models like Variational Autoencoders (VAEs) and Generative Adversarial Networks (GANs) to create entirely new molecules. These models analyze existing data to learn patterns and then use this knowledge to design compounds that meet specific criteria, such as high binding affinity or low toxicity.
- What are the biggest challenges in AI-driven drug discovery?
Key challenges include:- The need for high-quality, diverse datasets to train models.
- Ensuring AI models generalize well across different chemical spaces.
- Regulatory hurdles, particularly the lack of transparency in AI decision-making.
- The high computational cost of training and deploying advanced models.
- Can AI predict a drug’s safety and efficacy?
Yes, AI can predict a drug’s safety and efficacy by analyzing its Absorption, Distribution, Metabolism, Excretion, and Toxicity (ADMET) properties. These predictions help identify potential risks and optimize drug candidates before they enter costly clinical trials.
- Is AI already being used in real-world drug discovery?
Absolutely. AI has already accelerated the development of multiple drug candidates, some of which are now in clinical trials. For example, AI has been used to design kinase inhibitors for cancer and small molecules targeting rare diseases. Companies are increasingly partnering with AI-driven platforms to enhance their drug pipelines.
Conclusion: AI in Drug Design – Transforming the Future of Medicine
AI in drug design is more than a technological innovation; it’s a paradigm shift in how we approach medicine. By accelerating drug discovery, optimizing molecular properties, and exploring chemical spaces beyond human capability, AI is solving problems that once seemed insurmountable. Challenges remain, but they are dwarfed by the opportunities to save time, reduce costs, and bring effective treatments to patients faster.
The journey of AI-driven drug design is still in its early stages, but its trajectory is clear. As models become more advanced, datasets more diverse, and collaborations more widespread, we are witnessing the dawn of a new era in medicine. Whether through personalized treatments or the discovery of groundbreaking compounds, the future holds immense promise.
The possibilities are endless—and the impact will be profound. Stay informed and ready to embrace the next wave of innovation in drug design, because the future of medicine is already here.
References:
- Gangwal, A., & Lavecchia, A. (2024). Unleashing the power of generative AI in drug discovery. Drug Discovery Today, 29(6), 103992. https://doi.org/10.1016/j.drudis.2024.103992
- Zhung, W., Kim, H., & Kim, W. Y. (2024). 3D molecular generative framework for interaction-guided drug design. Nature Communications, 15, 2688. https://doi.org/10.1038/s41467-024-47011-2
- Pang, C., Qiao, J., Zeng, X., Zou, Q., & Wei, L. (2024). Deep generative models in de novo drug molecule generation. Journal of Chemical Information and Modeling, 64(7), 2174–2194. https://doi.org/10.1021/acs.jcim.3c01496