How do cells decide which genes to turn on and when? The answer lies in transcriptomics—the study of RNA, the molecules that carry genetic instructions from DNA to build proteins. Unlike epigenetics, which focuses on DNA modifications that regulate genes, transcriptomics looks directly at RNA to uncover which genes are active and how they respond to changes in the environment, development, or disease.
Understanding transcriptomics is essential for exploring how cells function, identifying disease mechanisms, and developing targeted therapies. In this guide, we’ll break down what transcriptomics is, the methods used to study it, and its wide-ranging applications in science and medicine. Whether you’re new to the topic or looking to deepen your knowledge, this is your starting point for understanding how transcriptome analysis is transforming biology.
The Foundations of Transcriptomics
Transcriptomics is a key branch of molecular biology that builds on the principles of the central dogma, which describes the flow of genetic information in living organisms. By studying RNA molecules, transcriptomics reveals how cells activate specific genes to respond to their environment, develop, or adapt during disease. To understand its importance today, it’s helpful to look at both its biological foundations and its historical development.
The Central Dogma: DNA to RNA to Protein
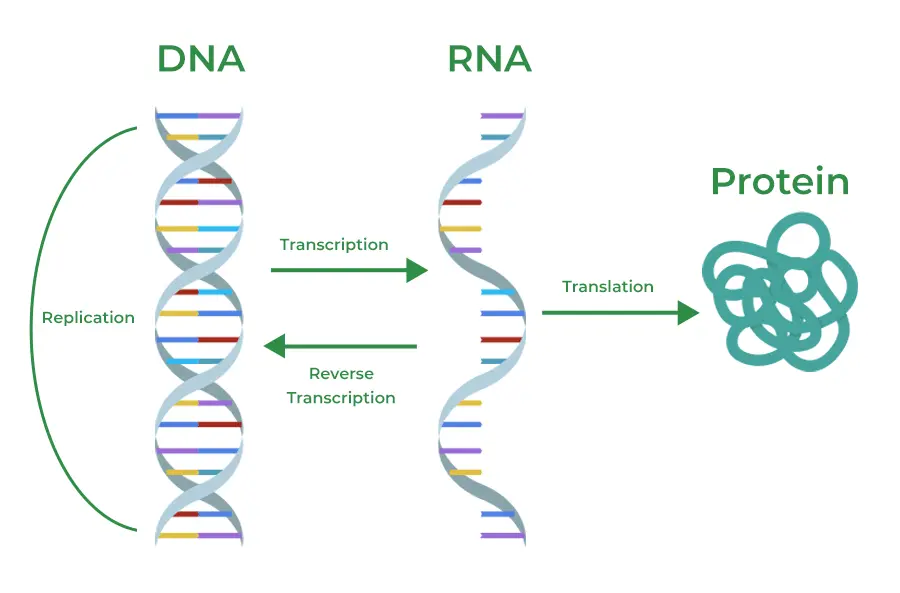
At the core of biology is the central dogma: DNA stores genetic information, RNA acts as the messenger, and proteins perform the cell’s functions. Genomics focuses on the DNA blueprint, but transcriptomics investigates the RNA molecules transcribed from DNA, offering insight into which genes are active at any given time.
This focus on RNA allows scientists to explore not just what a cell is capable of doing (as suggested by its genome) but what it is actively doing in response to specific conditions like stress, development, or disease.
What Is the Transcriptome?
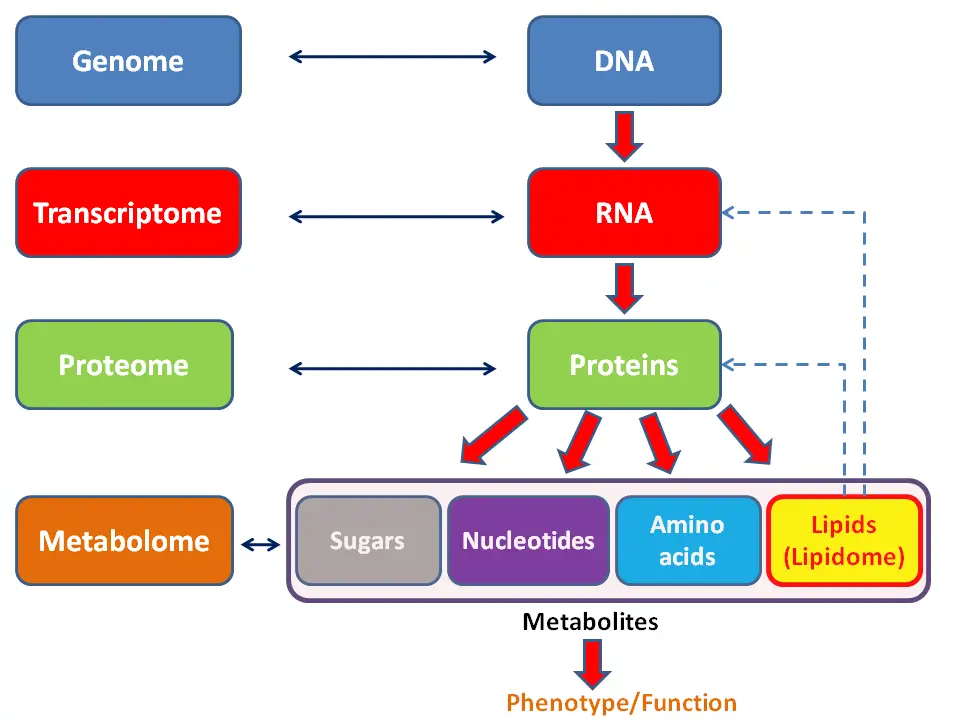
The transcriptome encompasses the full set of RNA molecules in a cell at any given moment, including:
- mRNA (messenger RNA): Carries the instructions for synthesizing proteins.
- tRNA (transfer RNA) and rRNA (ribosomal RNA): Work together to assemble proteins.
- Non-coding RNAs: Includes regulatory molecules like microRNAs and long non-coding RNAs, which control gene expression without coding for proteins.
Unlike the relatively stable genome, the transcriptome is dynamic, reflecting a cell’s real-time state. This makes transcriptomics particularly valuable for studying how cells adapt to changes or progress through stages of disease.
A Brief History of Transcriptomics
The study of RNA traces back to the mid-20th century, when the role of RNA as a messenger between DNA and proteins was first discovered. Early techniques, such as Northern blotting in the 1970s, allowed scientists to measure RNA levels for specific genes, but these methods were slow and limited in scope.
The real breakthrough came in the late 1990s and early 2000s with the development of DNA microarrays, which enabled the simultaneous measurement of thousands of gene transcripts. This innovation marked the beginning of large-scale transcriptome studies, providing the first glimpse of global gene expression patterns.
In the 2010s, RNA sequencing (RNA-Seq) revolutionized transcriptomics further. Unlike microarrays, which rely on predefined probes, RNA-Seq could capture all RNA molecules, including previously unknown transcripts. This method provided unprecedented resolution and has become the gold standard for transcriptome analysis today.
How Transcriptomics Differs from Other Omics
While related fields like genomics and proteomics also provide important insights, transcriptomics plays a distinct role:
- Genomics investigates the DNA blueprint, whereas transcriptomics reveals how that blueprint is used in real time.
- Epigenomics focuses on DNA modifications that regulate gene expression, while transcriptomics studies the RNA molecules that result from these regulatory changes.
- Proteomics analyzes the proteins produced by RNA, completing the flow of genetic information.
By linking DNA instructions to protein output, transcriptomics offers a real-time snapshot of cellular activity, bridging the gap between the static genome and the functional proteome.
Methods for Transcriptome Analysis
Transcriptome analysis involves two key stages: extracting RNA to study the molecules in a lab (in vitro) and analyzing the data to uncover meaningful patterns (in silico). Two widely used technologies—RNA sequencing (RNA-Seq) and microarrays—form the backbone of transcriptomics research. While RNA-Seq is considered the gold standard today, microarrays remain a valuable option in specific scenarios, offering benefits that newer technologies sometimes cannot match. Let’s explore how these methods work and what they bring to the table.
RNA Sequencing (RNA-Seq)
Extracting RNA and Sequencing (In Vitro)
The RNA-Seq process begins with isolating RNA from biological samples, such as tissues, cells, or even blood. Since RNA is fragile, researchers take care to preserve its quality during extraction. Once isolated, the RNA is reverse-transcribed into complementary DNA (cDNA), a more stable form suitable for sequencing.
The cDNA is then fragmented into smaller pieces, and adapters are added to create sequencing libraries. These libraries are loaded onto high-throughput sequencing platforms, such as Illumina or PacBio, which read the fragments and produce raw data as millions of short sequence reads.
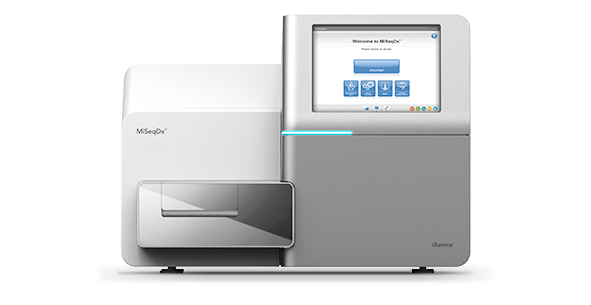
RNA-Seq is powerful because it captures a wide range of RNA types, including coding and non-coding RNAs. Its high sensitivity allows for the detection of rare transcripts and subtle changes in gene expression.
Analyzing RNA-Seq Output (In Silico)
Once sequencing is complete, computational analysis transforms raw data into actionable insights. This process involves four main steps:
- Quality Control: Tools like FastQC are used to evaluate the quality of sequencing reads, ensuring accurate downstream analysis. Low-quality data is filtered out to avoid errors.
- Alignment: Reads are mapped to a reference genome or transcriptome using software such as STAR or HISAT2. For organisms without reference genomes, de novo assembly tools like Trinity help reconstruct the transcriptome.
- Quantification: Gene and transcript abundance are measured using tools like Salmon or featureCounts, which estimate the expression levels of each RNA molecule.
- Differential Expression Analysis: Using software such as DESeq2 or EdgeR, researchers identify genes that are significantly upregulated or downregulated between experimental conditions.
These steps require robust computational infrastructure and bioinformatics expertise, but they enable a detailed view of gene activity, from common pathways to rare events.
Read more about Bulk RNA-Seq Data Analysis
Microarray Technology
Extracting RNA and Hybridizing (In Vitro)
Microarray technology, one of the earliest methods for transcriptome analysis, remains an important tool for specific applications. After RNA is extracted from the sample, it is either directly labeled with fluorescent dyes or converted to cDNA for labeling. This labeled RNA is hybridized to a microarray chip containing thousands of DNA probes designed to match specific genes. When complementary RNA binds to these probes, fluorescence signals indicate the presence and quantity of the corresponding gene transcripts.
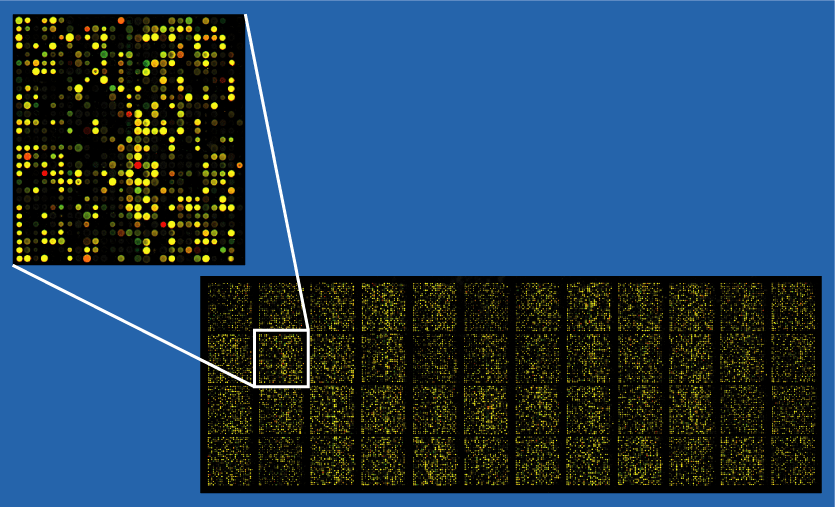
Although microarrays lack the flexibility of RNA-Seq, they offer consistent results when working with well-annotated genomes. Their simplicity makes them an attractive option for researchers focusing on targeted analyses.
Analyzing Microarray Output (In Silico)
The computational pipeline for microarray data is straightforward compared to RNA-Seq, making it accessible for smaller labs:
- Preprocessing: Background correction and normalization ensure that the fluorescence signals accurately reflect gene expression.
- Probe Mapping: Each signal is associated with a specific gene, allowing researchers to quantify expression levels.
- Statistical Analysis: Using tools like limma, researchers identify genes with significant changes in expression across experimental conditions.
- Visualization: Results are visualized using heatmaps or clustering techniques to identify patterns and relationships.
Comparing RNA-Seq and Microarrays
While RNA-Seq has largely overtaken microarrays in terms of versatility and sensitivity, microarrays still offer distinct advantages for certain research needs. They are less expensive and simpler to analyze, making them ideal for studies with large sample sizes or when working with well-characterized organisms. Additionally, microarrays have a well-established track record, with decades of use providing a wealth of standardized protocols and robust validation.
On the other hand, RNA-Seq is better suited for exploratory studies where researchers need to detect novel transcripts, analyze alternative splicing, or study organisms without reference genomes. Its dynamic range and ability to capture a wider array of RNA molecules provide deeper insights into complex biological systems.
Both technologies play a critical role in transcriptomics, and the choice between them often depends on the specific goals, budget, and resources of a study. Understanding these tools equips researchers to select the right approach for their needs and maximize the impact of their work.
Applications of Transcriptome Analysis
Transcriptome analysis offers you powerful tools to study how cells function, respond to their environment, and contribute to complex processes like disease and development. By focusing on RNA, you can uncover dynamic gene activity that provides insights into health, evolution, and agriculture. Let’s explore how you can apply transcriptomics to your research or studies.
1. Use Transcriptomics to Understand Disease Mechanisms
With transcriptome analysis, you can compare RNA profiles between healthy and diseased tissues to pinpoint genes involved in specific conditions. This helps you identify the molecular pathways driving diseases and develop targeted interventions.
- In Cancer: Analyze which genes are overactive in tumors and discover new drug targets. For instance, RNA-Seq allows you to identify gene mutations or alternative splicing events linked to cancer progression.
- In Neurodegenerative Diseases: Use transcriptomics to study how gene expression changes disrupt brain function in conditions like Alzheimer’s. You can also find potential biomarkers for earlier diagnosis.
- In Infectious Diseases: Investigate how pathogens like bacteria or viruses affect host cells. Transcriptomics helps you track immune responses and improve vaccine development.
2. Explore Gene Activity in Developmental Biology
Transcriptomics allows you to study how gene expression changes as organisms grow and develop. This is especially valuable for understanding the complex stages of cell differentiation and tissue formation.
- If you’re researching embryonic development, you can map how specific genes guide the formation of organs.
- In stem cell studies, transcriptome analysis helps you identify which genes activate when a stem cell transforms into a specialized cell type. This knowledge is key for advancing regenerative medicine.
3. Personalize Treatments with Transcriptomics
You can use transcriptome analysis to tailor medical treatments to individual patients. By studying a patient’s unique RNA profile, you can identify the therapies most likely to work for them.
- For cancer patients, transcriptomics helps you determine which genes are active in a tumor, guiding your choice of targeted therapies.
- In autoimmune diseases, analyze gene expression patterns to predict how patients will respond to treatments like biologics. This approach minimizes trial and error, improving outcomes.
4. Investigate Evolutionary Biology
Transcriptomics can help you understand how species adapt and evolve over time by comparing gene expression across different organisms or populations.
- You can study how gene activity changes in response to environmental pressures, like extreme temperatures or nutrient scarcity.
- By comparing RNA profiles between species, you can uncover which genes contribute to unique traits, such as faster growth or better survival strategies.
5. Advance Agriculture with Transcriptomics
If you’re working in agriculture, transcriptomics provides tools to improve crop yields, resilience, and quality. By studying plant responses to stress, pests, or poor soil, you can develop strategies to enhance productivity.
- Use RNA-Seq to identify genes that make crops more resistant to drought or disease.
- In livestock, apply transcriptomics to improve breeding programs by understanding the genetic basis of health and efficiency.
Transcriptome analysis provides answers to some of the most important questions in science and medicine. By exploring how genes are expressed in health, disease, and the environment, researchers can make discoveries that improve treatments, support conservation, and advance our understanding of life itself.
Future Advancements in Transcriptomics
The future of transcriptomics holds exciting possibilities for you to explore. New tools and techniques are making it easier to uncover detailed insights into gene expression. By adopting these advancements, you can study cells with greater precision, uncover hidden patterns, and drive impactful research.
1. Single-Cell Transcriptomics: Exploring Cellular Diversity
With single-cell RNA sequencing (scRNA-Seq), you can analyze gene expression at the level of individual cells. This technique helps you uncover cellular diversity and identify rare cell types that bulk RNA-Seq might miss. For example, in cancer research, you can use scRNA-Seq to study how different cells in a tumor respond to treatments. In immunology, you can map how immune cells fight infections or contribute to autoimmune diseases.
Single-cell technologies are becoming more accessible, so now is the time to incorporate them into your research to understand complex biological systems better.
2. Spatial Transcriptomics: Mapping Gene Expression in 3D
Spatial transcriptomics lets you see exactly where genes are active within tissues. This technology combines RNA analysis with imaging, giving you the ability to map gene expression to specific locations. For instance, you can study how genes are expressed across different regions of the brain in Alzheimer’s disease or how cells coordinate during tissue repair.
By adding spatial context to your research, you can uncover how cells interact with each other and their environment, leading to deeper biological insights.
3. Multi-Omics Integration: Connecting the Dots
You don’t have to stop at RNA. With multi-omics approaches, you can combine transcriptomics with genomics, proteomics, and metabolomics to get a full picture of cellular processes. For example, you can connect genetic mutations to RNA changes, protein functions, and metabolic pathways, helping you understand diseases more comprehensively.
Tools for integrating omics data are improving rapidly. Start exploring these methods to uncover connections that would otherwise remain hidden.
4. AI and Machine Learning in Transcriptomics
AI and machine learning are becoming essential for handling the large datasets generated by transcriptomics. You can use these tools to identify patterns, predict gene functions, and speed up your analysis. For example, machine learning can help you find biomarkers for disease or predict how cells respond to treatments based on their RNA profiles.
With AI, you can streamline your workflow and focus more on interpreting results rather than managing data.
5. Democratization of Transcriptomics
New technologies are making transcriptomics more affordable and easier to use. Portable RNA sequencing devices, like nanopore-based tools, allow you to conduct transcriptomics analysis in smaller labs or field settings. Open-source bioinformatics software also lowers barriers, letting you analyze data without needing expensive tools.
Take advantage of these accessible options to start your transcriptomics projects, no matter the size of your lab or budget.
FAQs About Transcriptome Analysis
- What is transcriptome analysis, and why is it important?
Transcriptome analysis studies the RNA molecules in a cell to understand which genes are active under specific conditions. It is important because it provides insights into how cells function, respond to changes, and contribute to health and disease. - How does RNA sequencing (RNA-Seq) work?
RNA-Seq involves isolating RNA from a sample, converting it to complementary DNA (cDNA), and sequencing the cDNA fragments using high-throughput technologies. This generates data that can be analyzed to measure gene expression levels, identify novel transcripts, and explore alternative splicing. - What is the difference between RNA-Seq and microarrays?
RNA-Seq uses sequencing to analyze all RNA molecules, providing high sensitivity and the ability to detect novel transcripts. Microarrays rely on pre-designed probes for specific genes, making them less flexible but more cost-effective for well-characterized genomes. - How is transcriptome analysis used in personalized medicine?
Transcriptome analysis helps tailor treatments by identifying gene expression patterns unique to an individual. For example, it can guide cancer treatments by revealing which genes are active in a tumor, helping doctors choose targeted therapies. - What are the main challenges in transcriptomics?
Challenges include handling large and complex datasets, ensuring reproducibility across studies, and managing the high costs of sequencing and computational analysis. Advances in AI and more affordable technologies are helping address these issues.
Conclusion: The Promise of Transcriptome Analysis
Transcriptome analysis helps us understand how genes are expressed and how cells behave in various conditions. By studying RNA, researchers gain insight into cellular activities, advancing fields like personalized medicine, disease research, and agriculture.
New technologies, such as single-cell and spatial transcriptomics, now allow scientists to examine gene expression in unprecedented detail. These tools uncover hidden details about cells and tissues, transforming our ability to address complex biological questions.
For students and researchers, transcriptomics provides the opportunity to explore life at the molecular level. Whether studying diseases or improving crops, these insights pave the way for meaningful discoveries. As the field evolves, its potential to shape science and medicine will continue to grow.
References
- Stahl, F., Hitzmann, B., Mutz, K., Landgrebe, D., Lübbecke, M., Kasper, C., Walter, J., & Scheper, T. (2012). Transcriptome analysis. Advances in biochemical engineering/biotechnology, 127, 1–25. https://doi.org/10.1007/10_2011_102
- Blumenberg, M. (2019). Introductory Chapter: Transcriptome Analysis. IntechOpen. doi: 10.5772/intechopen.85980